Module 2 - Handwriting Recognition
The goal of the text recognition module was to automatically transcribe images of historical handwriting into machine-readable text, which is provided to the information retrieval module.
This module receives text line images as input and automatically creates a machine-readable transcription. In order to train the module for a specific script and language, several prerequisites must be met:
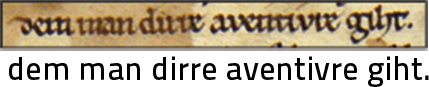
Training sample for automatic transcription
- A number of learning samples must be provided by human experts in form of text line images together with their machine-readable transcription. They are used to train character appearance models, which become more robust the more learning samples are provided.
- A vocabulary is needed for the language, preferably together with inflection rules, common abbreviations, and other spelling variants. In contrast to OCR for printed documents, recognition is performed at word level and hence a set of valid words is required to transcribe a text line image.
- If available, a large electronic text corpus can provide useful information for the recognition. Besides an extraction of vocabulary words, such a corpus can be used to build statistical language modelsthat estimate the probability of co-occurrences for consecutive words.
A semi-automatic procedure was developed to label the handwriting images of the IAM HistDB [C10.3]. Automatic transcription systems have been developed based on state-of-the-art methods for sequence recognition taking into account statistical language models [C09.2, C09.2].
Novel methods for keyword spotting have been devised [C10.4, C10.7]. Keyword spotting has not been emphasized in the research plan but it has turned out to be one of the central topics of interest in the field. The HisDoc methods have achieved some of the best results worldwide and are published in two articles in top journals [J1, J2]. Also, one of the research papers [C10.4] has received a Best Scientific Paper Award at ICPR, one of the leading conferences in pattern recognition. In the third year, we have introduced a new problem statement, that is, how to align existing transcriptions with document images if the text and the image do not perfectly correspond with each other. Two possible solutions have been proposed [C11.3, C11.4].
The main aim of the module was to provide flexible and robust methods for text recognition. Based on machine learning, the HisDoc methods are very flexible and can be adapted without much effort to different scripts and languages. Exemplary robustness results include a promising word accuracy of about 93 % for Gothic script and Old German language [Cn1]. All aims could be reached and with respect to keyword spotting and transcription alignment we could even go a step further than the research plan.
- IAM HistDB
- Module 1 - Document Image Analysis
- Module 3 - Information Retrieval
- Integrated System - Full Processing Chain
Publications
Thesis
[T1] A. Fischer. Handwriting Recognition in Historical Documents. PhD thesis, University of Bern, March 2012.
Book Chapters
[B1] A. Fischer, V. Frinken, and H. Bunke. Application of hidden Markov models for handwriting recognition. Handbook of Statistics, 31:421–442, 2012. 10.1016/B978-0-444-53859-8.00017-5
Journal Articles
[J1] A. Fischer, A. Keller, V. Frinken, and H. Bunke. Lexicon-free handwritten word spotting using character HMMs. Pattern Recognition Letters, 33(7):934–942, 2012. 10.1016/j.patrec.2011.09.009
[J2] V. Frinken, A. Fischer, R. Manmatha, and H. Bunke. A novel word spotting method based on recurrent neural networks. IEEE Trans. PAMI, 34(2):211–224, 2012. 10.1109/TPAMI.2011.113
Conference Papers, peer-reviewed
2012
[C12.1] A. Fischer, H. Bunke, N. Naji, J. Savoy, M. Baechler, and R. Ingold. HisDoc: Historical Document Analysis, Recognition, and Retrieval. In Digital Humanities, Book of Abstracts, pp. 94–97, 2012. Digital Humanities-Link
[C12.2] V. Frinken, M. Baumgartner, A. Fischer, and H. Bunke. Semi-Supervised Learning for Cursive Handwriting Recognition using Keyword Spotting. In Proc. 13th Int. Conf. on Frontiers in Handwriting Recognition, pp. 49–54, 2012. 10.1109/ICFHR.2012.268
[C12.3] V. Frinken, F. Zamora-Martinez, S. España-Boquera, M. J. Castro-Bleda, A. Fischer, and H. Bunke. Long-Short Term Memory Neural Networks Language Modeling for Handwriting Recognition. In Proc. 21st Int. Conf. on Pattern Recognition, pp. 701–704, 2012. IEEE-Link
2011
[C11.2] A. Fischer and H. Bunke. Character prototype selection for handwriting recognition in historical documents with graph similarity features. In Proc. 19th European Signal Processing Conference, pp. 1435–1439, 2011. Eurasip-Link
[C11.3] A. Fischer, E. Indermühle, V. Frinken, and H. Bunke. HMM-based alignment of inaccurate transcriptions for historical documents. In Proc. 11th Int. Conf. on Document Analysis and Recognition, pp. 53–57, 2011. 10.1109/ICDAR.2011.20
[C11.4] A. Fischer, V. Frinken, A. Fornés, and H. Bunke. Transcription alignment of Latin manuscripts using hidden Markov models. In Proc. 1st Int. Workshop on Historical Document Imaging and Processing, pp. 29–36, 2011. 10.1145/2037342.2037348
[C11.5] A. Fornés, V. Frinken, A. Fischer, J. Almazán, G. Jackson, and H. Bunke. A keyword spotting approach using blurred shape model-based descriptors. In Proc. 1st Int. Workshop on Historical Document Imaging and Processing, pp. 83–90, 2011. 10.1145/2037342.2037356
[C11.6] V. Frinken, A. Fischer, H. Bunke, and A. Fornés. Co-training for handwritten word recognition. In Proc. 11th Int. Conf. on Document Analysis and Recognition, pp. 314–318, 2011. 10.1109/ICDAR.2011.71
[C11.7] V. Frinken, A. Fischer, and H. Bunke. Improving handwritten keyword spotting with self-training. In Proc. 26th Symposium on Applied Computing, pp. 838–843, 2011. 10.1145/1982185.1982368
2010
[C10.3] A. Fischer, E. Indermühle, H. Bunke, G. Viehhauser, and M. Stolz. Ground truth creation for handwriting recognition in historical documents. In Proc. 9th Int. Workshop on Document Analysis Systems, pp. 3–10, 2010. 10.1145/1815330.1815331
[C10.4] A. Fischer, A. Keller, V. Frinken, and H. Bunke. HMM-based word spotting in handwritten documents using subword models. In Proc. 20th Int. Conf. on Pattern Recognition, pp. 3416–3419, 2010. 10.1109/ICPR.2010.834
[C10.5] A. Fischer, K. Riesen, and H. Bunke. Graph similarity features for HMM-based handwriting recognition in historical documents. In Proc. 12th Int. Conf. on Frontiers in Handwriting Recognition, pp. 253–258, 2010. 10.1109/ICFHR.2010.47
[C10.6] V. Frinken, A. Fischer, and H. Bunke. Combining neural networks to improve performance of handwritten keyword spotting. In Proc. 9th Int. Workshop on Multiple Classifier Systems, pp. 215–224, 2010. 10.1007/978-3-642-12127-2_22
[C10.7] V. Frinken, A. Fischer, and H. Bunke. A novel word spotting algorithm using bidirectional long short-term memory neural networks. In Proc. 4th Int. Workshop on Artificial Neural Networks in Pattern Recognition, pp. 185–196, 2010. 10.1007/978-3-642-12159-3_17
[C10.8] V. Frinken, A. Fischer, H. Bunke, and R. Manmatha. Adapting BLSTM neural network based keyword spotting trained on modern data to historical documents. In Proc. 12th Int. Conf. on Frontiers in Handwriting Recognition, pp. 352–257, 2010. 10.1109/ICFHR.2010.61
2009
[C09.1] A. Fischer and H. Bunke. Kernel PCA for HMM-based cursive handwriting recognition. In Proc. 13th Int. Conf. on Computer Analysis of Images and Patters, pp. 181–188, 2009. 10.1007/978-3-642-03767-2_22
[C09.2] A. Fischer, M. Wüthrich, M. Liwicki, V. Frinken, H. Bunke, G. Viehhauser, and M. Stolz. Automatic transcription of handwritten medieval documents. In Proc. 15th Int. Conf. on Virtual Systems and Multimedia, pp. 137–142, 2009. 10.1109/VSMM.2009.26
[C09.3] V. Frinken, T. Peter, A. Fischer, H. Bunke, T.-M.-T. Do, and T. Artieres. Improved handwriting recognition by combining two forms of hidden Markov models and a recurrent neural network. In Proc. 13th Int. Conf. on Computer Analysis of Images and Patterns, pp. 189–196, 2009. 10.1007/978-3-642-03767-2_23
[C09.4] M. Wüthrich, M. Liwicki, A. Fischer, E. Indermühle, H. Bunke, G. Viehhauser, and M. Stolz. Language model integration for the recognition of handwritten medieval documents. In Proc. 10th Int. Conf. on Document Analysis and Recognition, pp. 211–215, 2009. 10.1109/ICDAR.2009.17
Conference Papers, not peer-reviewed
[Cn1] A. Fischer, H. Bunke, N. Naji, J. Savoy, M. Baechler, and R. Ingold. The HisDoc Project. Automatic Analysis, Recognition, and Retrieval of Handwritten Historical Documents for Digital Libraries. In Proc. InterNational and InterDisciplinary Aspects of Scholarly Editing, in print, Bern, 2012. Link