HisDoc 2.0 Project Description
HisDoc 2.0 will address documents with complex layouts and several scribes – documents which have been circuited by the DIA research community so far. Existing approaches rather focus on subtasks, such as layout analysis, text line segmentation, writer identification, or text recognition. These naturally interrelated tasks are customarily treated independently. Furthermore, the approaches presume laboratory conditions, i.e., assumptions about the nature of the input are common practice. These assumptions include, e.g. high-quality separation of back- and foreground – a problem that is only partially solved, (manually) pre-segmented text line images, or pre-segmented text of only one scribe. Given a complex document with one or more main text bodies, annotations, embellishments, miniatures, etc., traditional methods will fail since several different challenges have to be met simultaneously. Reliable script analysis and text localization, for example, are mutually dependent: scribe identification relies on exact segmentation of homogeneous text regions on a page, which in presence of various kinds of scripts or writing styles then again depends on the ability of discriminating scripts.
Following this argumentation and the fact that the DIA community has produced a huge amount of contributions for subtasks of DIA, with HisDoc 2.0 we want to move forward to work on problems composed of several tasks. We start with the integration of text localization, script discrimination, and scribe identification into a holistic approach in order to obtain a flexible, robust, and generic approach for historical documents with complex layouts. Flexibility in this context means that the system can be adapted to documents of different sources and styles without great effort; robustness refers to correct results; generic means the method is not restricted to a certain kind of document. The second focus of the project is the incorporation of existing expert knowledge into DIA approaches by extracting data from semantic descriptions created by experts. A long-term goal of this research is to automatically translate results generated by DIA methods into human-readable interpretations, which can be used to enhance existing semantic descriptions and assist human experts.
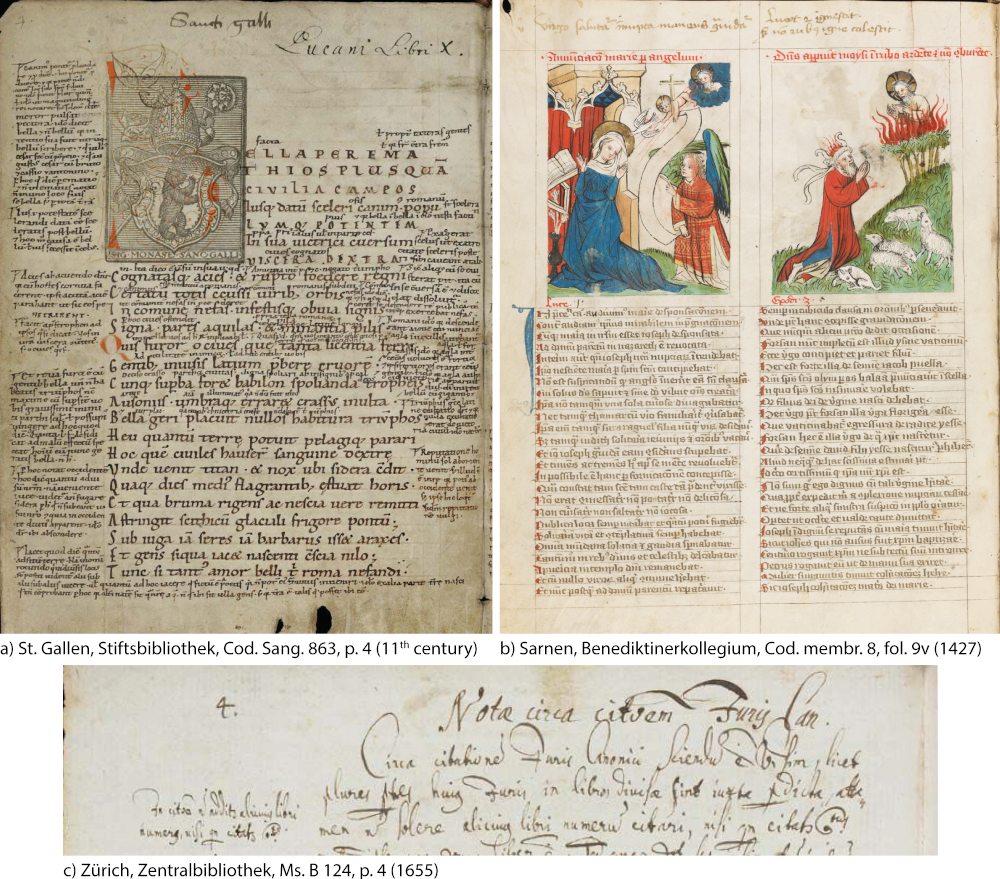
HisDoc 2.0 in detail
The aim of HisDoc 2.0 is to move forward from solving DIA (sub)tasks towards sub problems using an integrated approach which combines the tasks of text localization, script analysis, and semantic information for historical documents. The figure below summarizes and describes the difference between the common practice in state-of-the-art methods and our proposition.
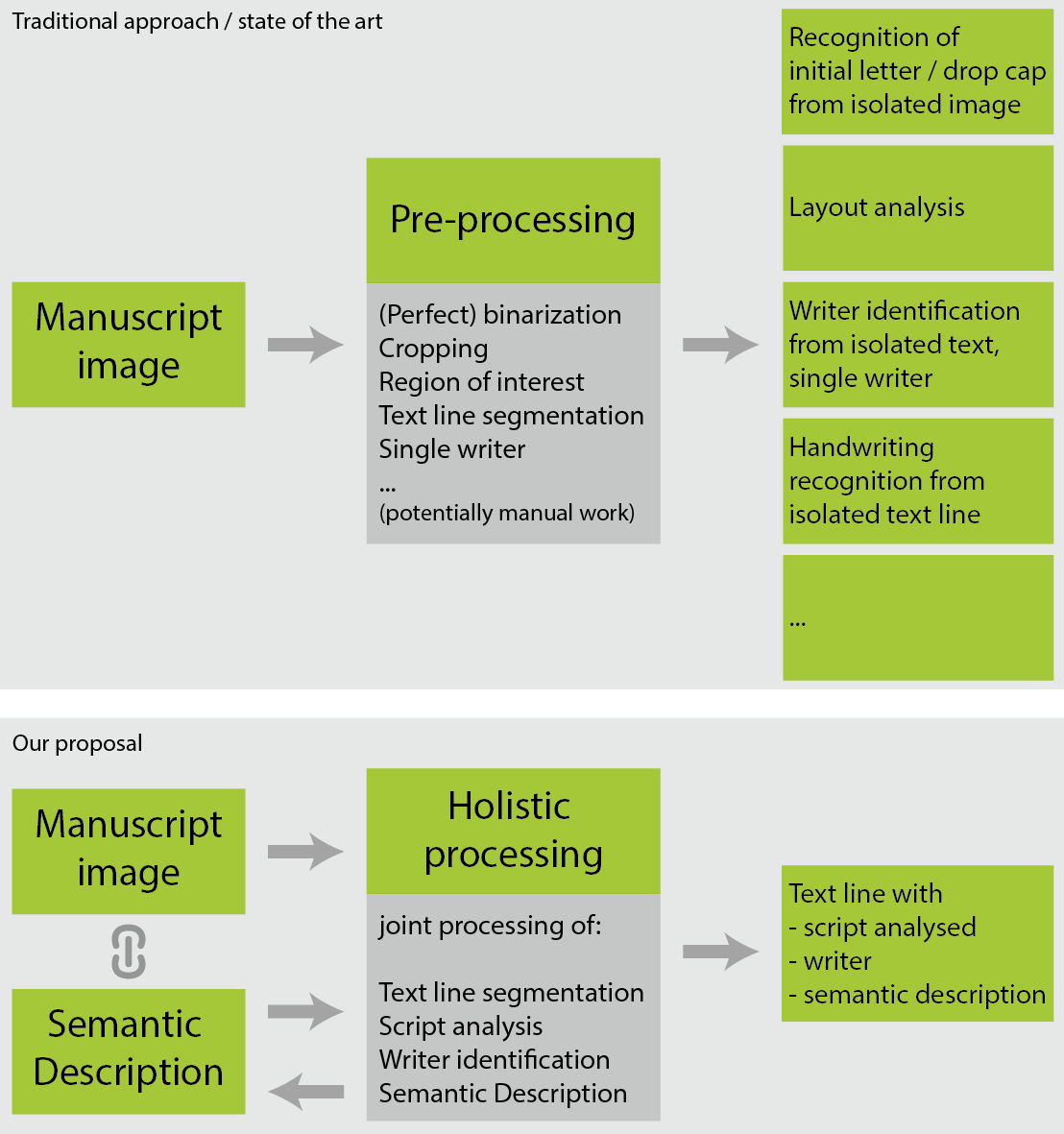
Text Localization
Text localization detects positions of text regions within a page, where the desired outcome is a set of segmented text lines. We will analyze methods for text localization, and furthermore propose an algorithm independent of assumptions about the layout (such as script, locations, orientations, numbers, and kinds of text entities, and relationships between them), and capable of handling complex layouts.
Script Discrimination
Script discrimination refers to detecting script changes occurring within a document. We address this issue by unsupervised clustering of uniform textual regions according to their visual similarity, i.e., discriminating scripts of unknown type and number. The aim consists just in grouping scripts or writing styles having similar properties, and not in assigning a specific scribe or script family. While features enhancing slight variations between different handwriting are sought for scribe identification, more general features capable of capturing larger variations are needed for script discrimination since a rather coarse decision whether or not a region is of the same script is sought for. Thus, we might rely on a more general subset of features used for scribe identification in this module, and add further features.
Scribe identification
We regard scribe identification as a more specific task of script analysis, i.e., rather than matching against a database of known writers, we will identify the number of scribes, the position of a scribe change, or different annotations by the same reader in one manuscript, or a specific part of a manuscript, respectively. Additionally to the analysis of existing features, we want to study the applicability of interest points to segment script primitives – for both script analysis tasks. They are capable of describing parts of different sizes, and can be applied at different granularity, i.e., capturing a range of details of handwriting, from small parts up to whole characters and character composites.
Combination
We want to combine the three modules of text segmentation, script discrimination, and scribe identification into one holistic approach. Three possible fusion methods are conceivable for the integration of text localization and script discrimination: sequential processing, where first script discrimination is done, and then results are included in text localization defining regions within which text lines can be concatenated; joint processing, which includes script discrimination in text line segmentation; and an iterative approach. Fig. 3illustrates the process of knowledge transfer between modules. Knowing text locations, script analysis can be accomplished either on text lines or text blocks. Information generated in script discrimination helps distinguishing different text blocks and facilitates the analysis in scribe identification. Furthermore, we can generate statistical information about handwriting in the text segmentation module, which can be incorporated in the script analysis process.
Semantic Data
The second major topic of HisDoc 2.0 will be dedicated to semantics. Databases of document collections published online are predominantly annotated with textual descriptions in natural language which poses the challenge of transforming them into machine-readable format. While some structure in form of XML exists, the containing textual descriptions (e.g. “Textura von zwei Händen”) are not normalized in terminology and content. Furthermore, varying quality and level of details between and within databases exist since different cataloging projects refer to different guidelines and focus on different details. The first step in this task is to define an ontology for the semantic description of historical documents. Therefore we will build upon existing database designs. Together with scholars in the humanities interested in the scope of the HisDoc and HisDoc 2.0 projects we will enhance these descriptions by adding axioms for the inference of new knowledge.
The crucial step of deriving computer understandable information from existing textual descriptions will be tackled as follows. Existing structured data will be directly used; making use of unstructured information, however, is not straight forward. We will use state-of-the-art natural language processing tools to extract information from the textual descriptions, i.e., we will identify entities defined in the ontology and automatically derive relations between the instances. The resulting semantic information will enable further automatic processing of the catalog entries in the future.
This text is an excerpt of the article
A. Garz, N. Eichenberger, M. Liwicki, and R. Ingold. HisDoc 2.0: Toward Computer-Assisted Paleography, Manuscript Cultures, vol. 7, pp. 19–28, 2015.
PDF